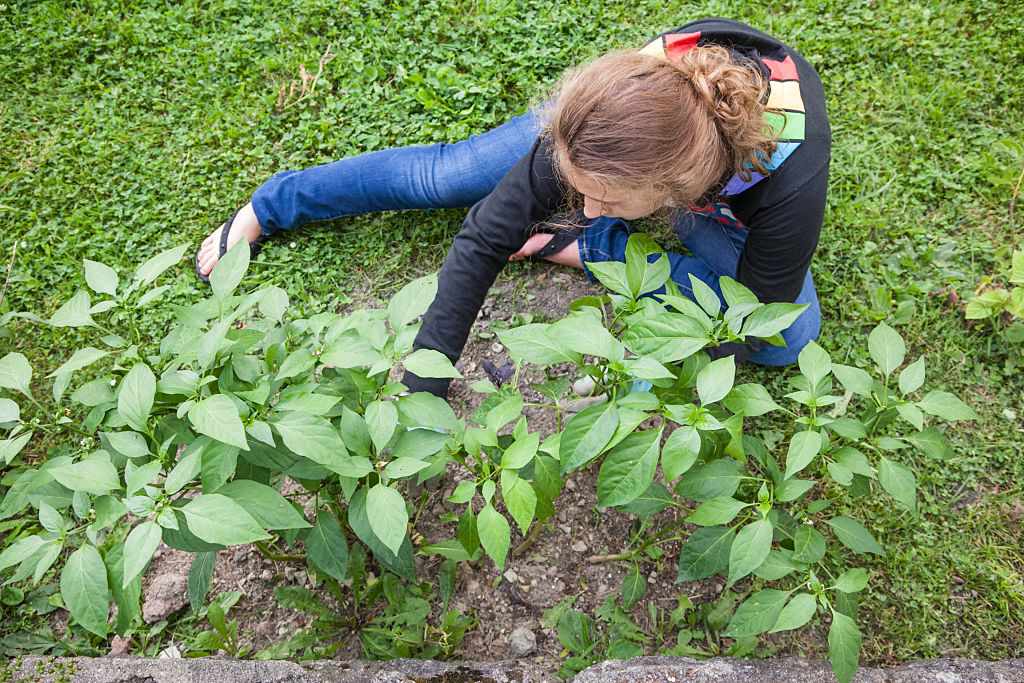
Unlocking the Future: 12 Key Developments in Data Science delves into the rapidly evolving field of data science, where technological advancements and innovative breakthroughs are shaping the way we analyze and interpret data.
This article explores the latest trends in big data, data analytics, predictive analytics, data visualization, machine learning, and more.
With a focus on technical precision and analytical insights, this piece offers valuable information for professionals seeking to navigate the ever-expanding world of data science.
Big Data Advancements
The emergence of big data has revolutionized the field of data science, enabling organizations to analyze vast amounts of information to gain valuable insights and make informed decisions.
One of the key advancements in this field is big data storage. With the exponential growth of data, traditional storage systems were no longer sufficient to handle the volume and velocity of data. As a result, new technologies and architectures have been developed to efficiently store and manage big data.
These advancements include distributed file systems, such as Hadoop Distributed File System (HDFS), which allows for the storage of large datasets across multiple machines, ensuring scalability and fault tolerance.
Additionally, advancements in data processing have played a crucial role in handling big data. Technologies like MapReduce have revolutionized data processing by allowing parallel and distributed processing of large datasets, enabling faster and more efficient analysis.
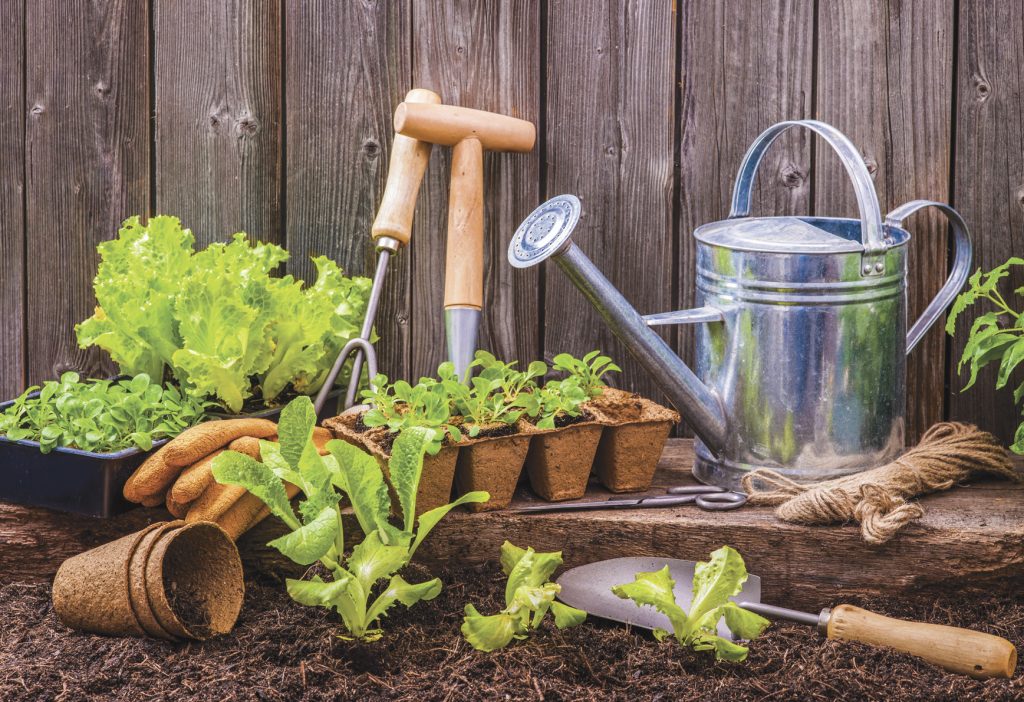
Data Analytics Breakthroughs
A significant number of breakthroughs have been made in data analytics, revolutionizing the field of data science. These advancements have greatly expanded the capabilities of data analytics applications, allowing organizations to harness the power of data-driven insights like never before.
With the increasing availability of big data, data analytics has become an essential tool for businesses to make informed decisions and gain a competitive edge. Sophisticated algorithms and machine learning techniques have enabled analysts to uncover hidden patterns and correlations within large datasets, leading to more accurate predictions and improved decision-making.
Furthermore, advancements in data visualization have made it easier for stakeholders to understand complex data sets and derive actionable insights.
Predictive Analytics Innovations
Building upon the breakthroughs in data analytics, predictive analytics has emerged as a powerful tool for organizations to anticipate future trends and outcomes. By utilizing advanced predictive modeling techniques and real-time data analysis, organizations can gain valuable insights that enable them to make informed decisions and stay ahead in a rapidly changing world.
Here are four key innovations in predictive analytics:
- Machine learning algorithms: These algorithms enable computers to learn from data and make predictions without being explicitly programmed. They are capable of handling large and complex datasets, allowing organizations to uncover hidden patterns and trends.
- Prescriptive analytics: This branch of predictive analytics not only predicts future outcomes but also provides recommendations on the actions to be taken to achieve desired outcomes. It helps organizations optimize their decision-making process.
- Automated predictive modeling: This innovation automates the process of building predictive models, making it faster and more efficient. It reduces the need for manual intervention and enables organizations to scale their predictive analytics capabilities.
- Real-time predictive analytics: With the increasing availability of real-time data, organizations can now perform predictive analytics on the fly. This allows for immediate decision-making and faster response to changing market conditions.
These predictive analytics innovations empower organizations to unlock the potential of data and make accurate predictions that drive growth and success.
Cutting-Edge Data Visualization Techniques
Cutting-edge data visualization techniques have revolutionized the way we present and analyze data. Interactive data storytelling allows users to engage with the data and explore different perspectives, providing a more immersive and interactive experience.
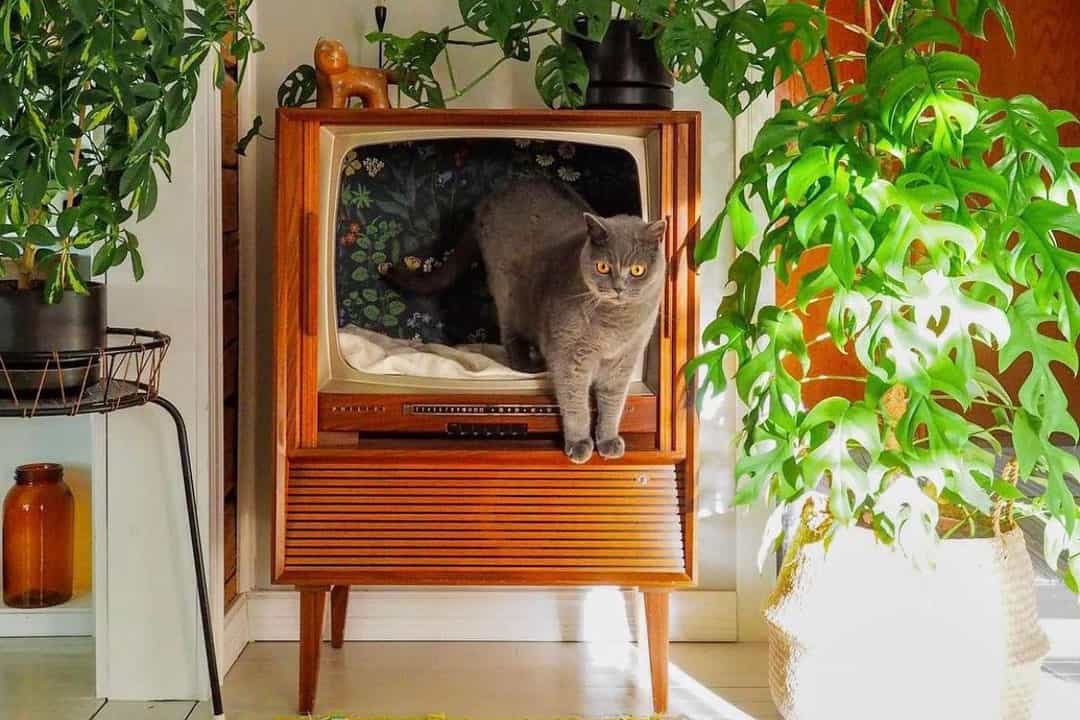
Additionally, the integration of immersive virtual reality technology has opened up new possibilities for visualizing complex datasets, enabling users to step into virtual worlds and gain a deeper understanding of the information at hand.
These advancements in data visualization techniques have the potential to greatly enhance data analysis and decision-making processes.
Interactive Data Storytelling
In the realm of data science, the evolution of interactive data storytelling has revolutionized the way information is presented and understood. This innovative approach combines data-driven journalism and interactive data visualization to create engaging narratives that empower users to explore and interpret data on their own terms.
Here are four key developments in interactive data storytelling:
- Enhanced User Interaction: Interactive data storytelling allows users to interact with data visualizations, enabling them to drill down into specific details, filter information, and gain deeper insights.
- Dynamic Data Updates: With interactive data storytelling, users can access real-time data updates, ensuring that the information presented is always current and relevant.
- Multi-dimensional Visualization: Through interactive data storytelling, users can explore data from multiple perspectives, enabling them to uncover patterns, trends, and relationships that may have been previously overlooked.
- Engaging Narrative Structures: Interactive data storytelling employs compelling narratives to present data in a more engaging and accessible way, making complex information more understandable and memorable.
Immersive Virtual Reality
Immersive virtual reality is revolutionizing data visualization techniques in the field of data science. With the advancements in technology, virtual reality applications are now being utilized to create immersive and interactive data visualizations. These virtual reality simulations provide a unique and engaging way to explore and analyze complex datasets.
By using virtual reality, data scientists can visualize large datasets in three dimensions, allowing for better understanding and interpretation of the data. Users can navigate through the data, manipulate it, and gain insights in ways that were not possible before. This level of immersion enables a more intuitive and natural interaction with the data, leading to enhanced decision-making and problem-solving capabilities.
Furthermore, virtual reality applications also offer the opportunity to collaborate and communicate with others in a virtual environment. This can be particularly useful for teams working on data-driven projects, as it allows for real-time collaboration and exploration of the data together.
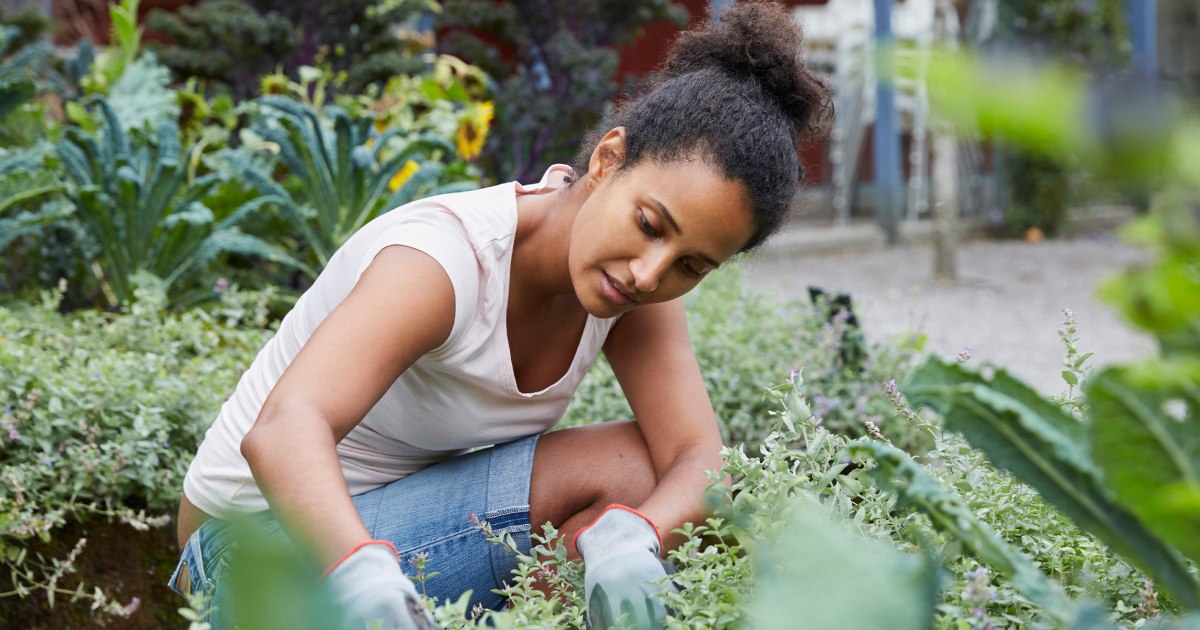
Machine Learning Applications in Data Science
One of the most significant advancements in data science is the emergence of numerous machine learning applications. Machine learning algorithms have the ability to analyze vast amounts of data and make predictions or decisions based on patterns and trends. These applications have revolutionized various industries and have a wide range of real-world applications.
Here are four key areas where machine learning is making a significant impact:
- Healthcare: Machine learning algorithms can analyze medical records and identify patterns to predict diseases, improve diagnosis accuracy, and personalize treatment plans.
- Finance: Machine learning is used in fraud detection, credit scoring, and algorithmic trading to identify patterns and make data-driven decisions.
- Marketing: Machine learning algorithms help analyze customer behavior, predict customer preferences, and optimize marketing campaigns for better targeting and personalization.
- Autonomous vehicles: Machine learning is crucial in developing self-driving cars, enabling them to recognize objects, detect obstacles, and make real-time decisions.
While machine learning applications offer immense potential, ethical considerations around data privacy, bias, and transparency need to be addressed to ensure responsible and fair use of these technologies.
Data Science Impact on Business
Data science has a significant impact on business by enabling organizations to transform their data into valuable insights and actionable strategies.
By leveraging advanced analytical techniques, businesses can make more informed and data-driven decisions, leading to improved operational efficiency and cost savings.
Moreover, data science provides a competitive advantage by identifying patterns and trends that can help businesses identify new opportunities and stay ahead of the competition.
Business Data Transformation
Businesses are increasingly relying on data science to drive transformational changes, as evidenced by the growing frequency with which organizations are incorporating data-driven insights into their decision-making processes.
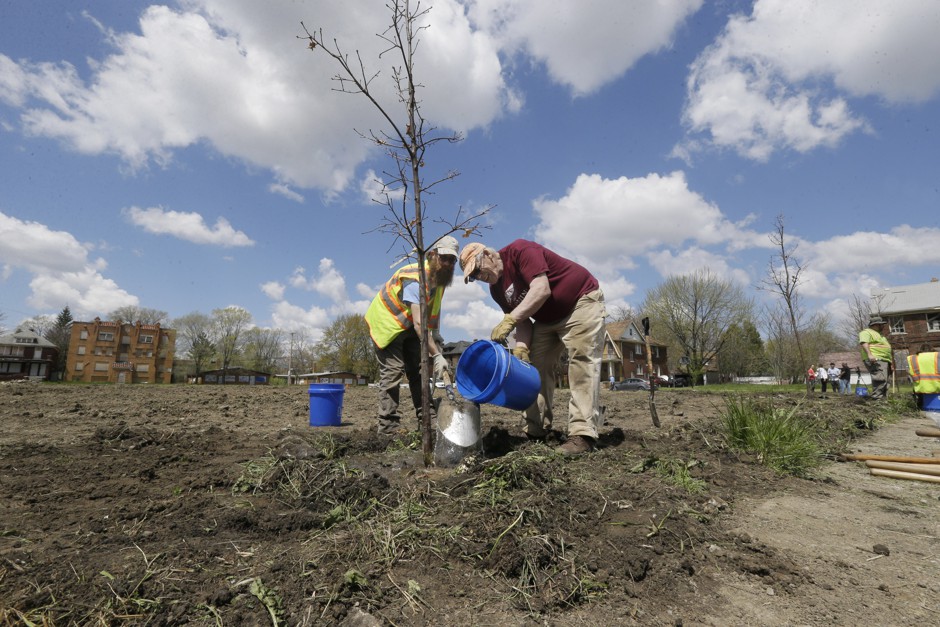
In the realm of business data transformation, several key developments are shaping the landscape:
- Business data optimization: With the help of data science, businesses can optimize their data management processes, ensuring that data is collected, stored, and analyzed efficiently and effectively.
- Data-driven marketing strategies: Data science enables businesses to develop marketing strategies based on data insights, allowing for targeted and personalized campaigns that deliver higher conversion rates.
- Predictive analytics: By leveraging data science techniques, businesses can predict future trends and customer behavior, enabling them to make proactive decisions and stay ahead of the competition.
- Operational efficiency: Data science helps businesses identify inefficiencies in their operations and processes, allowing for optimization and cost reduction.
These developments in business data transformation highlight the growing importance of data science in driving innovation and competitiveness in the business world.
Enhanced Decision-Making Process
As organizations embrace data science, it is imperative to recognize its transformative impact on the decision-making process within businesses.
Improved decision-making techniques through data-driven approaches have become a necessity in today's rapidly changing business landscape.
Data science enables businesses to make more informed and accurate decisions by leveraging the vast amounts of data available to them.
Traditional decision-making processes relied heavily on intuition and experience, which were often subjective and prone to biases.
However, with the advent of data science, organizations can now analyze large datasets, identify patterns, and extract valuable insights to make data-driven decisions.
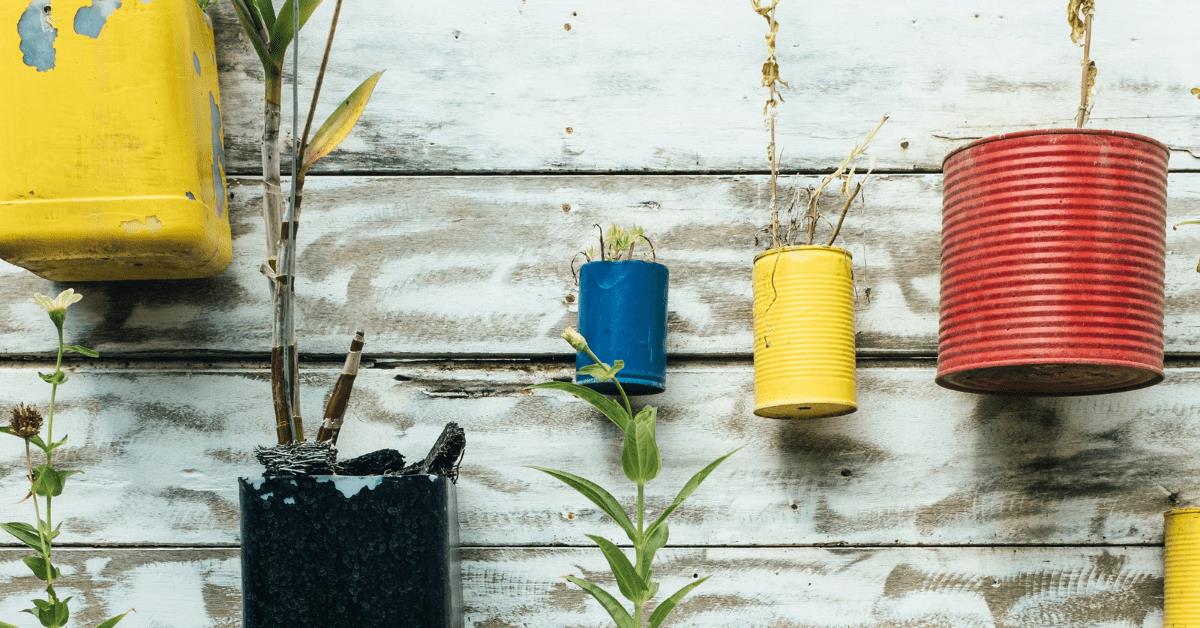
This approach ensures that decisions are based on objective data rather than personal opinions, leading to more effective and efficient outcomes.
Competitive Advantage Through Data
The utilization of data science provides organizations with a means to leverage data for a competitive edge in the business landscape. By harnessing the power of data, companies can make data-driven decisions and drive innovation in their operations.
Here are four ways data science can contribute to a competitive advantage:
- Data-driven decision making: Organizations can use data science techniques to analyze vast amounts of data and extract valuable insights. These insights enable businesses to make informed decisions based on evidence rather than intuition or guesswork.
- Improved customer understanding: Data science allows organizations to collect and analyze customer data, providing a deeper understanding of customer needs, preferences, and behaviors. This knowledge enables businesses to tailor their products and services to meet customer demands effectively.
- Enhanced operational efficiency: Data science can optimize processes and streamline operations by identifying inefficiencies, predicting maintenance needs, and automating tasks. This efficiency improvement leads to cost savings and increased productivity.
- Data-driven innovation: Data science can uncover new opportunities and drive innovation by identifying patterns, trends, and correlations in data. This enables organizations to develop new products, services, and business models that meet changing market demands.
Data Science Revolutionizing Healthcare
How is data science transforming the landscape of healthcare?
With the advent of healthcare analytics, data science is revolutionizing the way healthcare is delivered.
By analyzing vast amounts of patient data, data scientists are able to identify patterns and trends that can lead to improved outcomes and personalized medicine.
Healthcare analytics allows for the integration and analysis of diverse data sources, including electronic health records, genomic data, and wearable devices, to provide a more comprehensive view of a patient's health.
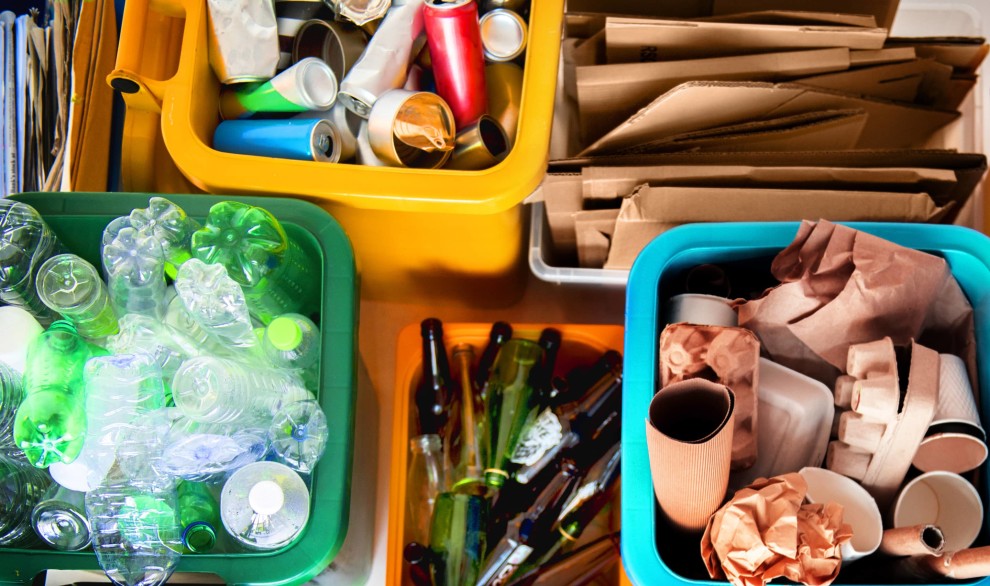
This enables healthcare providers to make more informed decisions, tailor treatment plans to individual patients, and predict potential health risks.
The application of data science in healthcare has the potential to greatly enhance patient care, improve efficiency, and ultimately save lives.
Data Science Transforming Finance
Data science is revolutionizing the financial industry through its ability to analyze large amounts of data and uncover valuable insights. Here are four ways data science is transforming finance:
- Risk management: Data science techniques, such as machine learning algorithms, can analyze past financial data to identify patterns and predict future risks. This helps financial institutions make informed decisions and mitigate potential losses.
- Fraud detection: By analyzing vast amounts of transactional data, data science can detect anomalies and patterns associated with fraudulent activities. This enables financial institutions to minimize losses and protect their customers.
- Customer segmentation and targeting: Data science in marketing allows financial institutions to understand customer behavior and preferences. This helps them tailor their marketing strategies and offers to specific customer segments, increasing customer satisfaction and loyalty.
- Logistics optimization: Data science in logistics helps financial institutions optimize their supply chain and reduce operational costs. By analyzing data on inventory levels, transportation routes, and demand patterns, they can make informed decisions to streamline their logistics processes.
Essential Data Science Tools
To successfully navigate the complex world of data science, professionals require top data science tools that enable them to extract valuable insights from vast amounts of data. These must-have data tools encompass a wide range of technologies, including programming languages like Python and R, data visualization tools such as Tableau and Power BI, and machine learning frameworks like TensorFlow and scikit-learn.
As data continues to grow in volume and complexity, having access to these essential tech for data becomes crucial for data scientists to drive informed decision-making and solve complex business problems.
Top Data Science Tools
As data science continues to advance, it is crucial to familiarize oneself with the top tools that are essential for this field. These tools enable data scientists to effectively analyze and interpret large amounts of data, extract meaningful insights, and build accurate predictive models.
Here are the top data science tools that every aspiring data scientist should be proficient in:
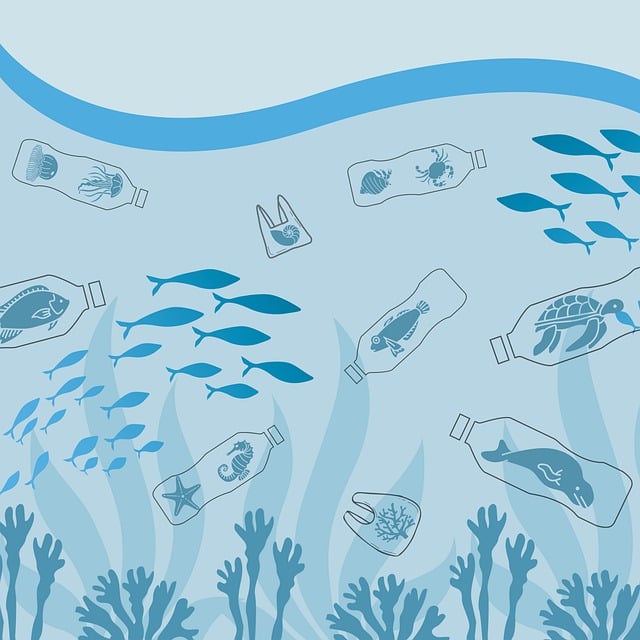
- Python and R: These are the most widely used data science programming languages, offering a wide range of libraries and frameworks for data manipulation, visualization, and machine learning.
- Apache Spark: This distributed computing framework is highly efficient for processing big data and running complex data analytics tasks.
- TensorFlow and PyTorch: These deep learning frameworks provide powerful tools for building and training neural networks, enabling advanced machine learning algorithms.
- Scikit-learn: This machine learning library in Python provides a comprehensive set of tools for various data science tasks, including classification, regression, clustering, and dimensionality reduction.
Must-Have Data Tools
A comprehensive arsenal of essential data science tools is necessary for any data scientist looking to excel in their field.
Data cleaning techniques play a crucial role in ensuring that the data is accurate, consistent, and reliable. These techniques involve identifying and correcting errors, handling missing values, and removing outliers.
Data visualization software, on the other hand, allows data scientists to present their findings in a visually appealing and easily understandable manner. This software enables the creation of charts, graphs, and interactive visualizations that facilitate the exploration and communication of insights derived from the data.
Essential Tech for Data
Utilizing essential data science tools is fundamental for unlocking the future of data analysis and decision making. In today's world, where data advancements are occurring at an unprecedented pace, having the right technology is crucial for success.
Here are four essential tech tools that are revolutionizing the field of data science:
- Machine Learning Algorithms: These algorithms enable computers to learn from data and make predictions or take actions without being explicitly programmed. They are essential for building intelligent systems that can analyze large datasets and extract valuable insights.
- Data Visualization Tools: These tools allow analysts to present complex data in a visually appealing and easily understandable format. They help in identifying patterns, trends, and outliers, enabling better decision making.
- Big Data Processing Platforms: With the exponential growth of data, traditional data processing tools are no longer sufficient. Big data processing platforms like Hadoop and Spark enable the processing and analysis of massive datasets, providing scalability and efficiency.
- Cloud Computing: Cloud computing platforms provide on-demand access to computing resources, allowing data scientists to store, process, and analyze data without the need for extensive infrastructure. Cloud platforms also offer collaboration capabilities and scalability, making them essential for modern data science workflows.
Ensuring Data Privacy in Data Science
To ensure data privacy in the field of data science, it is imperative that strict protocols and encryption measures be implemented.
One key aspect of protecting data privacy is data anonymization, which involves removing or encrypting personally identifiable information from datasets. By doing so, the risk of re-identification is minimized, ensuring that individual privacy is preserved.
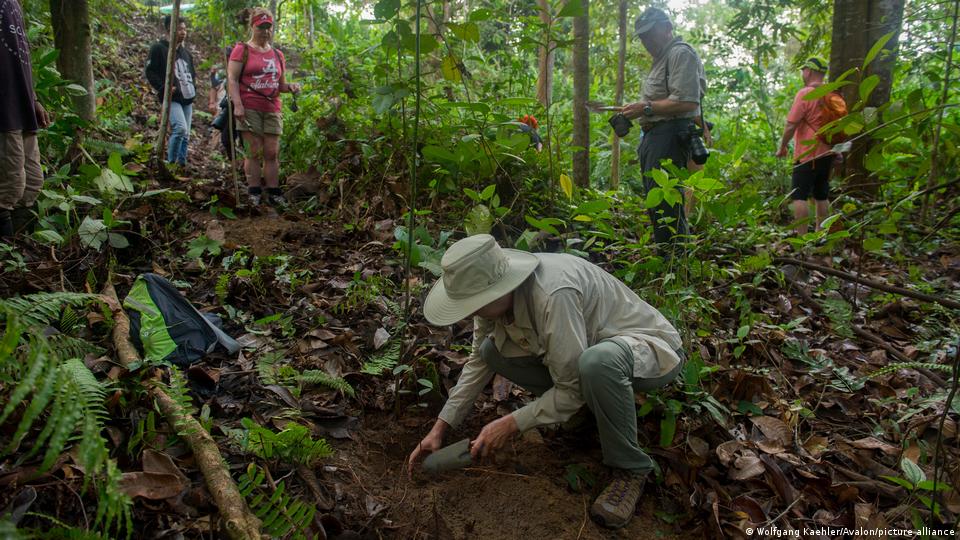
Additionally, data protection measures such as encryption and access controls should be implemented to safeguard sensitive information. Encryption ensures that data is encoded and can only be accessed by authorized entities with the proper decryption keys.
Access controls, on the other hand, restrict data access to authorized personnel, preventing unauthorized individuals from accessing or manipulating sensitive data.
Importance of Data Governance
How can data governance ensure the integrity and quality of data in the field of data science?
Data governance plays a crucial role in maintaining the importance of data quality and ensuring its integrity throughout the data science process. Here are four key ways in which data governance strategies contribute to the overall data quality:
- Standardization: Implementing standardized data governance policies and procedures ensures consistency and accuracy in data collection, storage, and analysis.
- Data validation: Data governance enables the establishment of validation rules and protocols to verify the accuracy, completeness, and reliability of data.
- Data cleansing: By implementing data governance strategies, organizations can identify and rectify data inconsistencies, errors, and redundancies, improving data quality.
- Data lineage tracking: Data governance provides a framework for tracking the origin, transformations, and movement of data, allowing data scientists to understand the lineage and reliability of the data being used.
Exciting Careers in Data Science
In the field of data science, there are numerous exciting career opportunities that allow professionals to explore and contribute to cutting-edge advancements in the field.
The demand for data scientists is at an all-time high, as organizations across industries recognize the value of data-driven insights.
From analyzing large datasets to developing machine learning models, data scientists play a crucial role in extracting actionable information from complex data.
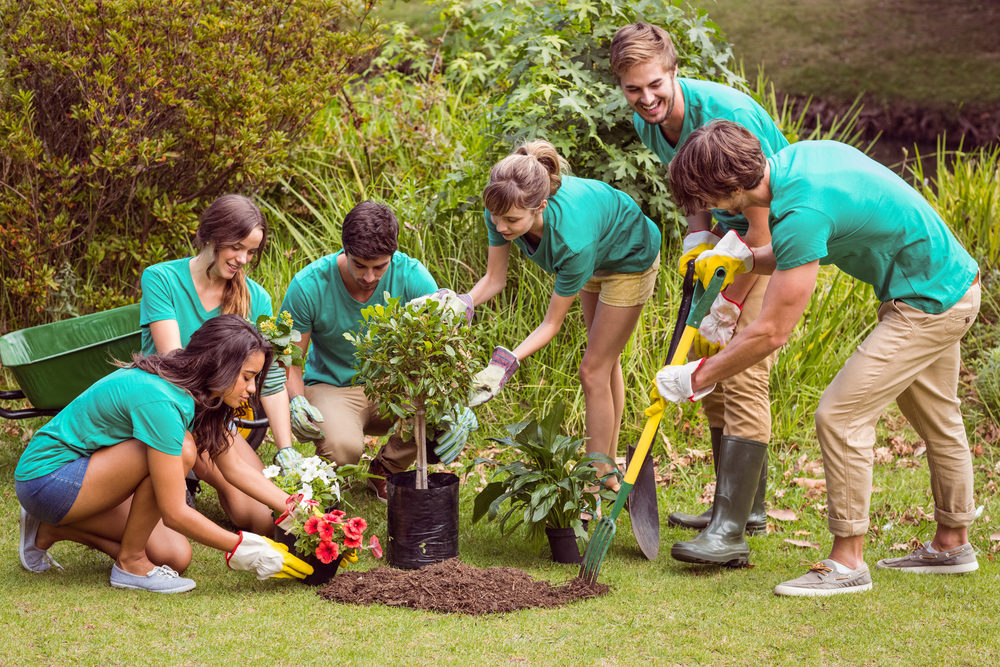
To succeed in this field, individuals need a strong foundation in mathematics, statistics, and programming, as well as a deep understanding of data science techniques and methodologies.
Additionally, effective communication and problem-solving skills are essential for translating data insights into meaningful business recommendations.
As data science continues to evolve, professionals with the right skills will have the opportunity to shape the future of industries and make a significant impact in their chosen career paths.
Frequently Asked Questions
What Are the Ethical Considerations in Data Science and How Are They Being Addressed?
Ethical implications in data science include issues of data privacy and protection. These concerns are being addressed through the implementation of strict regulations, transparency, and accountability measures to ensure the responsible and ethical use of data.
How Are Data Scientists Using Natural Language Processing in Their Work?
Data scientists are utilizing natural language processing (NLP) techniques to extract meaningful insights from large volumes of text data. NLP applications range from sentiment analysis to text summarization, enabling more effective decision-making and automation in various industries.
What Are the Challenges in Implementing Data Science Projects in Small Businesses?
The challenges in implementing data science projects in small businesses include limited resources, lack of data infrastructure, and the need for skilled personnel. These constraints require careful planning and prioritization to ensure successful implementation.
How Is Data Science Being Used in the Field of Environmental Sustainability?
Data science is being used in the field of environmental sustainability to analyze and interpret large volumes of data related to climate change, resource management, and conservation efforts. This enables informed decision-making and the development of sustainable solutions.
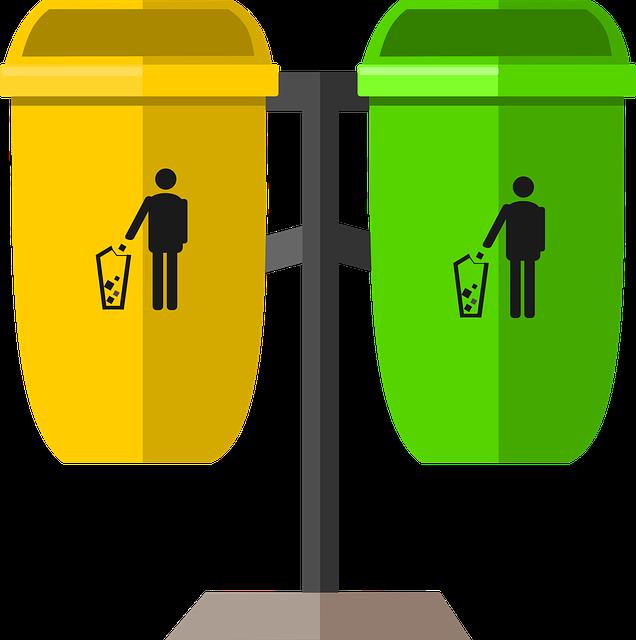
What Are the Limitations of Current Data Science Techniques and How Are Researchers Working to Overcome Them?
Researchers in data science are actively working to overcome the limitations of current techniques. Through advancements in algorithms, machine learning, and data preprocessing, they aim to enhance accuracy, scalability, and interpretability, paving the way for future developments in the field.